Intro
I spend a lot of time reading and thinking and talking about the future of AI in business and have come to a conclusion: we all better start creating AI-native infrastructure right now if we expect to succeed in the age of intelligence.
The way most people interact with generative AI today goes like this: open ChatGPT, give it a prompt, and it gives back an answer. This is called zero-shot prompting - you’re asking a large language model (LLM) to provide a response based on only a few keywords or ideas and it will use its existing knowledge to give you a response. It’s like asking a smart college student to write an essay for you all at once without using backspace or asking any follow up questions.
Essentially, the LLM is guessing what you want based on the perceived sentiment of your prompt and trying to match it to its vast knowledge base. Annoyingly, this is also why “prompt engineering” is a thing - just like with humans, the way you ask AI for stuff matters. But with some finesse, the results can be quite impressive.
However, the next wave of gen AI will feature something called “agents”. Not just chat interfaces, but actual task-performing assistants that operate autonomously. In fact, they’re already here, but largely still being pieced together by savvy hobbyists and not quite ready for commercial use.
In the coming months, we will also see much faster, more intelligent LLMs, with an increase in memory, and a growing populace ready to test them with real-world use cases. Put this all together and the set of tasks that artificial intelligence can perform will expand dramatically and lead us into a new era in business no longer exclusively operated by humans.
A New Intelligence stack
If today’s LLMs are like a brain, then think of these agents as adding arms and legs. Your zero-shot college student is about to graduate into a very promising young associate that will ask qualifying questions, conduct the necessary research, create the first draft, consider the weakest parts, make revisions, and present you with a much more polished response.
This is just like how humans work. We plan, we iterate, we reflect, and we improve until we arrive at the best possible solution.
Beyond self-reflection, agents will also interact directly with tools, like email, search engines, cloud storage, and SaaS platforms through the use of APIs. Tools are important because they don’t perform like LLMs. They are predictable, consistent, and hard coded to always produce the same output. They also already exist so we don’t need to build them from scratch.
Finally, agents will also be able to plan and collaborate just like us. They will be able to allocate resources and communicate directly with different LLMs. There will even be specialists and generalists just like us, with some agents fine-tuned for specific roles and responsibilities. For example, one agent might act like a CEO on Claude that interacts directly with another agent that’s trained to be a software engineer on Lllama. These multi-agent systems will actually chat with each other, have debates, test ideas, and reason with each other.
When this human-like reasoning process is performed by AI, we call this an agentic workflow, and when they arrive en masse the business world will never be the same again.
So what’s the catch? There is always a catch.
The catch is that almost everyone will have access to the same powerful tools, LLMs, and agentic workflows. With each wave, AI becomes more and more ubiquitous and accessible so brute force AI won’t be enough to stand out and outperform your competition.
Just like in a human-only business, execution is what matters most.
- In order to execute, we must delegate
- In order to delegate, we must communicate.
- In order to communicate, we must understand.
So what you should really be focusing on is not the level of intelligence, but the level of cognition.
The contextual layer
In order to maximize the value of these automated agents for your company, you need to build a “contextual layer” that they can interact with in order to understand your brand, your industry, and your competitive landscape. Otherwise, you’ll just get the same (albeit increasingly impressive) results as everyone else that just slaps the latest LLM on top of their tech stack and hopes their team can figure it out faster than the rest of market.
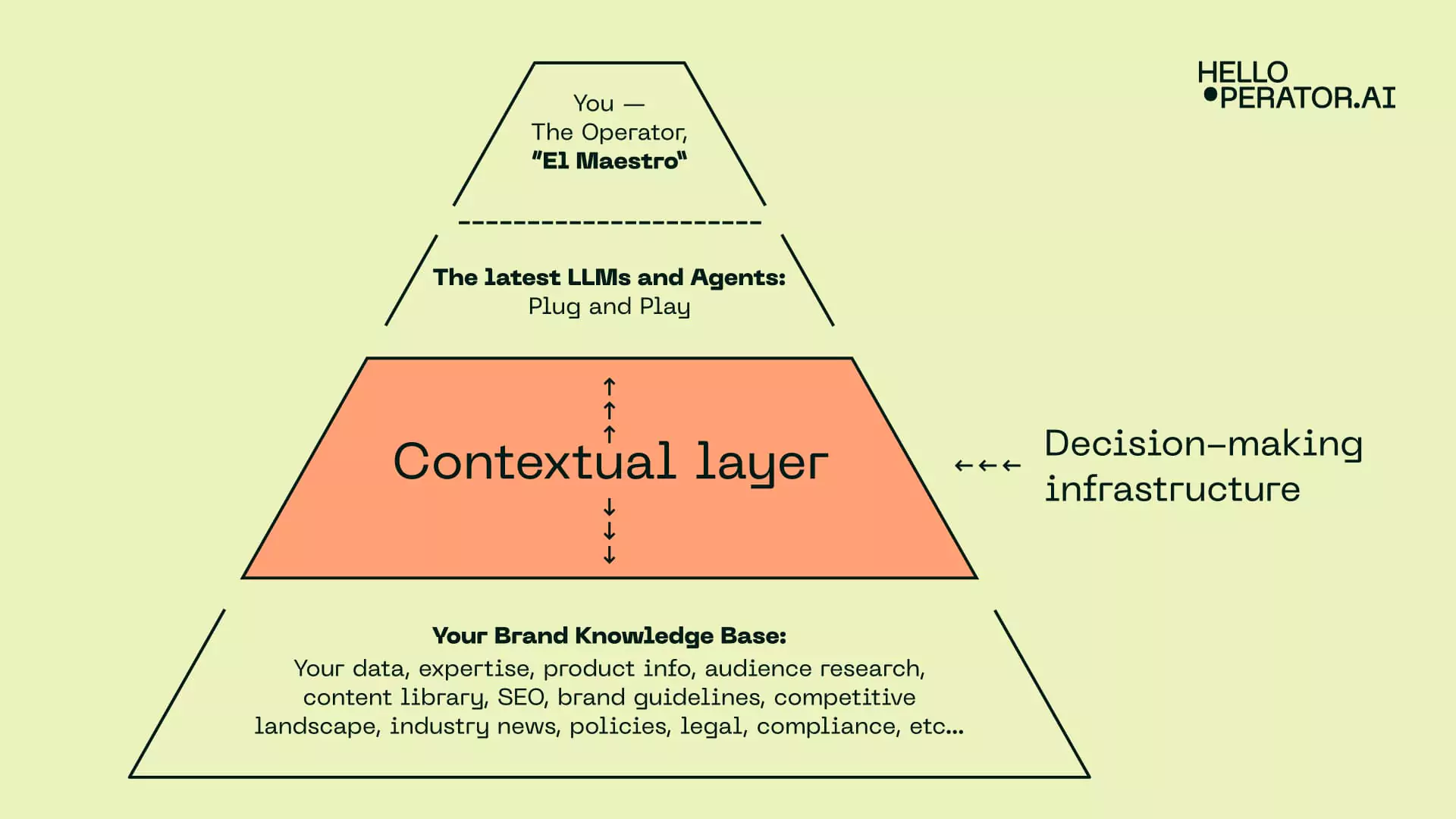
This contextual layer sits between your knowledge base and the agents and LLMs that are interacting with it. Not only must it connect all your key data points, but it must do so in a way that contextualizes the data with an understanding of your business. This creates a common ground for any agent or LLM to acquire knowledge, reason, make decisions, self-improve, and so on.
In other words, context is what enables actual cognition and pushes your AI agents beyond human intelligence mimicry into a coherent understanding of your business.
Sounds a bit like science-fiction doesn’t it? Well it is, for now. The current state of developing automated agents is still very messy and chaotic. But while agents might not seem so smart today, the smart money is betting on their rapid advancement and if you want to get ahead of it, you should start laying down these cognitive railroad tracks now.
Let’s look at an example:
AI-assisted content production
I’m a marketing guy, so let’s start with a straightforward process like content creation.
Here’s how our team is running AI-assisted content production processes today - imagine it like going through a car wash:

It’s essentially the same process for all content assets like case studies, white papers, videos, blog articles, landing pages, you get the idea.
Today, we’re involving gen AI in every stage of this production process as a co-pilot on a (very) short leash. We build custom GPTs for each stage of the content creation car wash.
We train these individual GPTs for:
- Brainstorming & idea generation
- Brief & outline creation
- Research & industry news
- Style, voice & tone
- Proofreading & grammar
- Localization & translation
- Schema markup & meta data
- Social snippets & video shorts
- Repurposing & recycling
For written content, we also refine each draft through the latest LLMs from Claude, Mistral, CoPilot, Llama and Gemini. With these tests, we’re learning which platforms perform best for different industries, languages, and content types. We test for inference (speed), token consumption (cost), ability to follow instructions (common sense), and hallucinations (accuracy). This allows us to match the right platform to the right task depending on its level of complexity, urgency, and importance to the business.
Up to this point, each car wash cycle is operating more or less in a vacuum and there’s direct human involvement in every stage. Since we’re paranoid about hallucinations and our clients value high quality, we’re still manually writing, editing, modifying, approving, and moving the assignment through each stage of the car wash. It’s just now we have some heavy duty AI assistance. Admittedly, it is cool, but it’s far from autonomous.
Furthermore, our content car wash is not retaining much of the knowledge from one assignment to the next and it’s not meaningfully improving over time. While AI is making us significantly better and faster at our jobs, it’s still a bit of a Mechanical Turk.
Agentic content production
Now imagine if this content car wash was able to run completely by itself, and:
- Generate ideas based on real-time shifts in demand.
- Align directly with your CRM data and seasonal sales cycles.
- Personalize interactive case studies by sector, persona, and stage of the funnel.
- Track your competitors and learn from their insights and results as well as your own.
- Search the industry for influencers and partners that are actively running relevant campaigns.
Most importantly, what if the outputs were primarily based on your brand’s proprietary knowledge base and running itself through the car wash several times? Each time it’s learning, optimizing and improving on itself before presenting you with the results.
Now you have a content engine that no one else can replicate.
This is what the future of gen AI looks like in the context of content creation and it’s the environment that we’re helping our clients prepare for to win market share.
But there’s no reason to stop at marketing. With the right infrastructure, these workflows can be applied to every aspect of your business, from hiring and training, to sales and customer service, to financial reporting and forecasting, to capex deployment and expansion.
Everything is self-optimizing. All options are considered, evaluated and presented in the context of what’s best for your business in real-time market conditions. Speed, meet quality. Adding autonomous agents to your core business processes will enable enhanced decision making abilities at every level, with human experts overseeing and making adjustments across your entire operation.
I want to be clear that we’re not advocating removing human oversight from any business process. We’re just rethinking the intelligence stack to include these new abilities and free up human creativity to spend more time on strategic oversight that orchestrates these processes instead of being cogs in the machine. Like anything else, agents will probably be underwhelming at first, but over time, you should increasingly be operating on your business rather than inside it.
Conclusion
So how can you best position your company for the next wave of LLMs and agents?
In my opinion, the companies that will get the most value from agentic workflows will be the ones that are most thorough and organized when it comes to documenting their business processes and systems.
After all, running a business is just a system optimization problem. You have finite resources. You put those resources into a system (ie: your business model) and hopefully more new resources are created than were expended.
Fortunately, AI is pretty good at optimizing systems, as long as it can understand them.
My advice, start getting your house in order now by documenting, updating, and organizing your company’s knowledge base so the next generation of LLMs and agents will have the context to efficiently understand your business, not just guess at how to best improve it.
And if you’re just starting out, create your business with an agentic mindset and document everything. It’s clearly the future and the future is bright.
____
This piece was written by AI.
Just kidding. But it was inspired by many conversations with founders, marketing leaders, and AI experts. Some are true believers and others are skeptics not impressed with all the hype. Most fall somewhere in between. We’re sharing our ideas, wisdom, workflows, tactics and relevant AI updates at HelloOperator.ai.
Join us and let us know your thoughts on the future of AI in business.
----
Resources and recommended reading:
- Self-Refine: Iterative Refinement with Self-Feedback, Madaan el al. (2023)
- Reflexion: Language Agents with Verbal Reinforcement Learning, Shinn el al. (2023)
- Communicative Agents for Software Development, Qian et al. (2023)
- What’s next for AI agentic workflows ft. Andrew Ng of AI Fund (2024)
- AutoGen: Enabling Next-Gen LLM Applications via Multi-Agent Conversation, Wu et al (2023)